On November 29 2022 I implored the tech world to bring on winter: We’re out of good ideas. I should have kept my mouth shut: The next day ChatGPT was unleashed on the public, and we haven’t paused for breath since. I believe we users need to contribute more to the debate and figure out where we stand, and what we stand for.
The mad rush to compete in this space means the big players are rolling their AIs out not only before they’re ready, but silly money is being thrown at startups promising exploitation of these tools. A massive land-grab is taking place, with little idea of the consequences and with the ink of some 155,000 tech layoff slips barely dry.
I wish I could be more sanguine. I’ve always loved technology, and I am absolutely bowled over by the latest iteration of ChatGPT, GPT-4. Everyone else has been writing about their experiences with it, so I won’t bore you with mine, but there’s no question we’re in Kansas anymore. This technology will change a lot. A LOT.
But we need to keep our eye on the ball. Some have called for a moratorium, which is at best naive and at worst giving the industry too much credit for a sense of responsibility. That’s not what is going on here. It’s money.
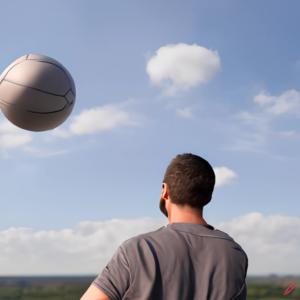
The ball we need to keep an eye on is market (and political, but we’ll leave for later) power, and we should be watching it carefully as it shifts. It doesn’t shift far, but it is shifting. We are not witnessing disruption in the sense that Clayton Christensen defined it, we’re seeing a further agglomeration of power from those lower down the pyramid to those at the top.
Peek behind the curtain of all this GPT magic, and what do we find?
There are, for sure, a lot of really bright people doing cutting-edge stuff. But behind them are thousands, possibly hundreds of thousands, of contract workers labelling and annotating the data that is fed into the software. The Transformer-type models we’re talking about are essentially trying to predict the next token (think ‘word’) in a document, drawing on data. That data has to be prepped for the algorithms and that means annotating, or labelling it.
So this process is automated? Actually no. The data still needs to annotated, to prepare it for the algorithms. The method involved is called “reinforcement learning from human feedback”, where model responses are ranked by quality, and then a reward model is trained to predict these rankings. As per the term, this is done by humans, and is a very labour-intensive process. This is how GPT-4 described it to me:
The process of collecting comparison data and ranking responses can be labor-intensive and time-consuming. By collaborating with outside contractors, organizations can scale their data collection efforts and obtain a diverse range of human feedback, which can help improve the performance of AI models.
This “collaboration” (clearly GPT-4 has a sense of humour) is done by contractors, “flexible contributors” or “ghost workers”. The biggest company doing this is Appen, which has on its books more than a million of them. After some protest those working on behalf of Google saw their rates rise to up to $14.50 an hour. Compare that to the average base salary of a Google employee of $125,000.
The data
And what is the data they’re annotating, exactly? What is in the datasets being used to train these massive language models is a mostly black box, since it’s considered commercially sensitive. Researchers from EleutherAI concluded that
Despite the proliferation of work exploring and documenting issues with datasets, no dataset intended to train massive language models has been seriously documented by its creators 1
But these aren’t quite the hallowed corpora you might imagine.
The data is for the most part the web. They have just been parcelled up into larger datasets, such as The Pile, an open source dataset of a (relatively measly 800 GB). And there’s MassiveText, 10.5 terabytes, which is private. (When I asked GPT-4 for a list of the biggest datasets, MT wasn’t included, because GPT-4’s data ends in September 2021, illustrating how new some of this stuff is.)
And what is this data, exactly? Well, it’s actually what you and I produce in our daily lives. It’s social media, webpages, news, Wikipedia pages, books, Youtube comments (and possibly transcribed content). Pretty much anything that we do online.
One paper2 estimated that up to half of the content in these so-called high quality datasets — high quality because they’re real sentences, with real context, etc — is user content scraped from the web. Books and scientific papers make up for up to 40%, with code, news, Wikipedia making up the rest. In other words, our musing, utterances, the journalism we write, the Wikipedia pages we tend: all are sucked into datasets that then, eventually, become the answers that ChatGPT or Google’s Bard spew out. Wikipedia, to give you an idea, weighs in at between 43 GB and 10 TB, depending on what you’re including.)
Unsurprisingly, there will inevitably be charges of plagiarism. My prediction, though, is that we’ll get better at identifying when GPT regurgitates existing material and tweaks it to try to hide it — it’ll be an escalating war of technology which will end in class lawsuits and significant legal hazard for some.
The other cost
So once the data is marked up, the algorithms need to do their work. And this is where things quickly get beyond the reach of scrappy startups. GPT-3, for example, is estimated to cost millions of dollars to train, and to run. And that’s just the processing. You also need the infrastructure.
Plugging GPT into Microsoft’s search engine Bing requires 20,000 8-GPU servers, meaning it would cost the company $4 billion. Reuters (hat-tip Gina Chua) quoted SemiAnalysis as calculating it would cost Google, sorry Alphabet, some $3 billion if they added ChatGPT-style AI to their search.
The scarcity
So where are we going with this? I’ve expressed elsewhere my concern that the biggest danger from these innovations is that they’ll be harnessed to manipulate — in other words, that the information they contain and the process they use to deliver it are best viewed as weapons of disinformation.
But just as likely, I believe, is that the competition currently underway will face constraints that in turn cause market players to turn to more drastic measures to remain competitive. In other words, that technology will evolve in the same way that search and Web 2.0 evolved — turning the user as much into a willing provider of valuable data as a consumer.
Here is a hint of what may come: The models themselves might be — possibly already have been — turned on our data that legal protections have worked hard to keep anonymous. Researchers from Romania and Greece used GPT to see whether they identify text of famous people from anonymous data. They found that in 58% of cases they could. Their conclusion:
[W]e believe that it is only a matter of time before organisations start using LLMs on their documents and realise that this way, not only can they get more visibility about their customers, but they can also deanonymise documents revealing information that would be impossible for them to do so.
Another concern is that GPT models are running out of source material — data. One paper estimates that what it calls ‘high-quality language data’ will be exhausted by 2027, if not earlier. This in spite of language datasets growing in size . The paper concludes:
If our assumptions are correct, data will become the main bottleneck for scaling ML models, and we might see a slow- down in AI progress as a result. 3
I’m sure something will come along to fix this. LLMs will become more efficient and require less data, or so-called synthetic data — data not derived from the real world, but from a virtual world — will develop to add to the sum of available datasets. (Gartner believes that 60% of all data used in the development of AI will be synthetic by next year.)
This might be fine, or it might not. The problem with synthetic data is that it’s not real. It’s not human and so while we, for all our imperfections, at least create a data exhaust that’s real, synthetic data is a simulation of that. And while it might work for programming autonomous driving, questions should be asked of its usefulness for training GPT and LLMs. This may create a premium for real, human, data that makes it impossible for those companies once committed to maintaining our privacy to resist selling it.
And another thing: the more we generate content through GPTs, the more that artificial content will start to appear in the data sets being used to build and advance GPTs. In other words, inhuman data becomes part of the food chain. Once these models rely on scraped data that itself is the product of AI, either synthetically created, or created as the result of us asking questions of (‘prompting’) the AI, then we’ll all be swimming in regurgitated AI-generated content. Given how frequently GPT-4 hallucinates when I use it, it will eventually become impossible to differentiate between something real and something artificial.
The usual suspects
Some final points: We are essentially in the hands of people who do not know what they have created. Literally. They cannot peer into the black box that is their creation, because like most of what calls itself AI, it’s a giant soup of knobs and sliders and wires that, when fed enough and given enough power, can do some useful stuff. Very useful. But we still don’t really know how it does this, and so neither do we know what other things it can do, and where its limits and weaknesses are.
In an excellent piece in Quanta, Stephen Ornes explores the unpredictable “emergent” abilities discovered within LLMs that reveal both extraordinary, undreamed of functionality, but also biases and inaccuracies. A growing list ranges from Hindu knowledge to detecting figures of speech. For now, no one knows whether this is a spontaneous new skill or a more plodding, chain-of-thought process. Ornes quotes computer scientist Ellie Pavlick as saying: “Since we don’t know how they work under the hood, we can’t say which of those things is happening.”
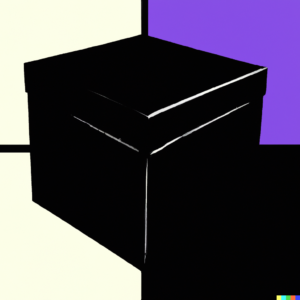
That’s one issue. Another is that the people who have created these tools are surprisingly poor in understanding how the rest of humanity might use, interact with, view these machines. Sam Altman, much of the brains behind OpenAI, told Lex Fridman in a recent interview that while “most other people say ‘him’ or ‘her’ he only used ‘it’ when referring to his AI progeny. “It’s really important,” he said, “that we try to explain, to educate people that this is a tool and not a creature.” Fridman, to his credit, pushed back, saying we shouldn’t draw hard lines. Altman’s admission is revealing: You might be forgiven for thinking that someone who has ‘raised’ an AI and seen it take flight would have built some sort of relationship with it.
While it might be reassuring that the likes of Altman don’t get overly connected to their offspring, it reveals a lack of imagination on his part about how ordinary users are likely to perceive it. We give inanimate machines names and assign them personalities — our cars, our boats — so it’s not hard to imagine a text- or voice-based UI which responds in intelligent sentences will quickly be assimilated as sentient creatures into our world.
The bottom line: we’re dealing with something that is a natural outgrowth of dominance by major tech companies which are able to leverage their computing heft, their expansive data lakes and their deep pockets into something that is both new and old: new because we’ve not seen a machine exhibit intelligence at this level before, and old because it’s the natural consequence of the internet we’ve created in the past decade or so. We’ve produced enough English-language content to provide fodder for these computing beasts and while there’s a bit of us in every response an LLM spits out, we have little say in how that data is being used, and little confidence our interests will be well served ahead of Mammon and, inevitably, national security.
This is not a brave new generation of upstarts improving life for ordinary folk and disrupting the existing hierarchy. It is a bunch of people who are smart enough to create something extraordinary, but with surprisingly little awareness of what their creation may take us. This isn’t about calling for a moratorium, it’s about the rest of us thinking seriously about our own position in this new food chain.
- The Pile: An 800GB Dataset of Diverse Text for Language Modeling, arXiv:2101.00027, 31 Dec 2020 ↩
- Will we run out of data? An analysis of the limits of scaling datasets in Machine Learning; arXiv:2211.04325v1 26 Oct 2022 ↩
- Man vs the machine: The Struggle for Effective Text Anonymisation in the Age of Large Language Models; arXiv:2303.12429v1, 22 Mar 2023 ↩